Residual RL
Can we train a more sample efficient RL policy using a better action representation?
This is a RL class project with Xiangyun Meng and Mohit Shridhar
In this work, we explored if we can improve human demonstrations in simulation using residual reinforcement learning. Our key insight is that by reducing action space via PCA, we can dramatically reduce the high sampling complexity, while preserving behavior features from the demonstrations, which leads to a more sample efficient and smooth trajectory refinement.
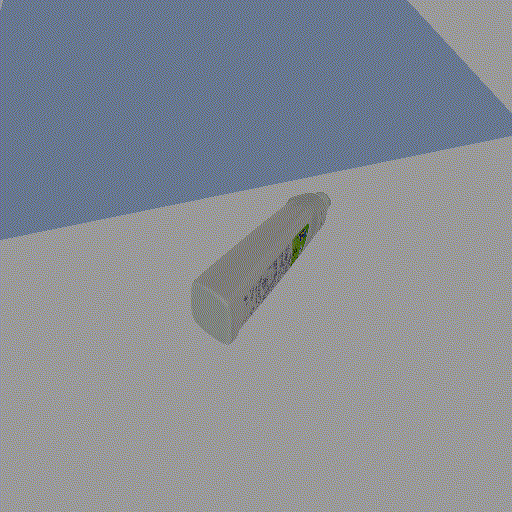
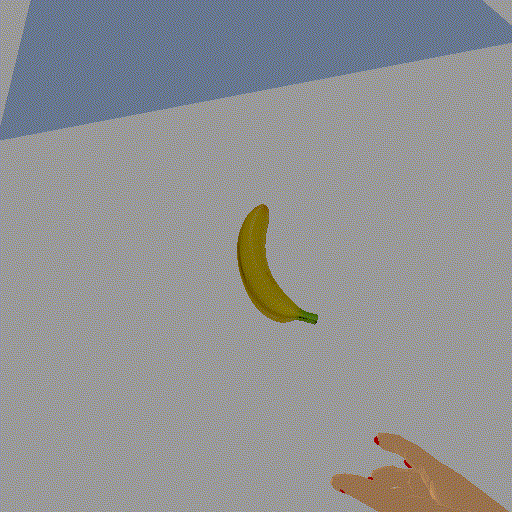
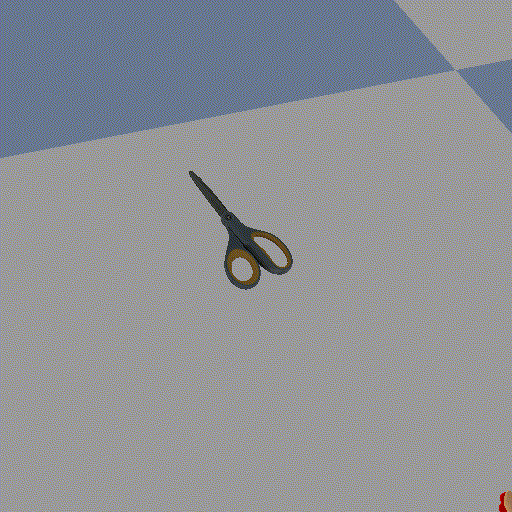
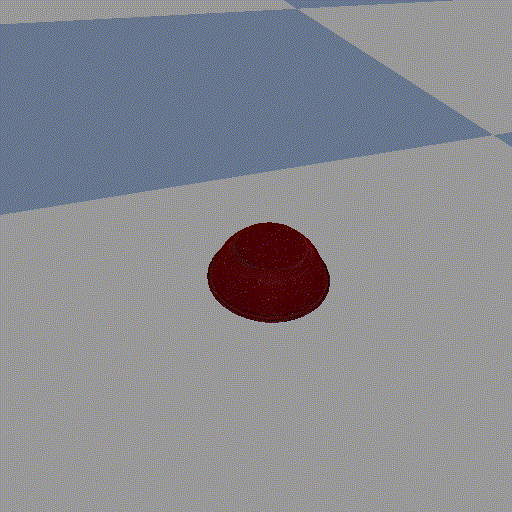
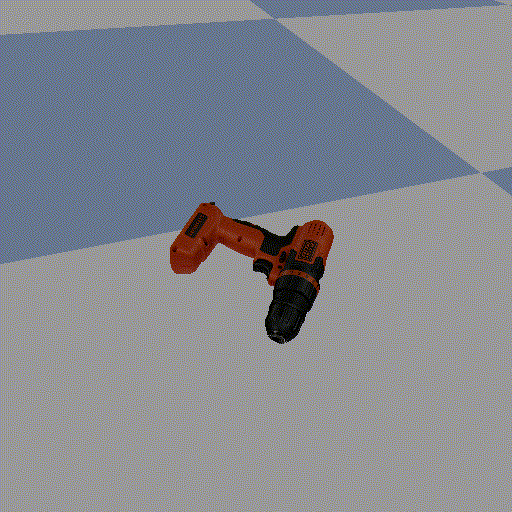
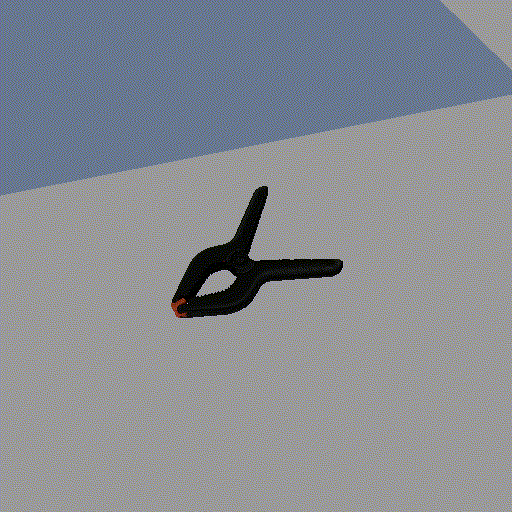
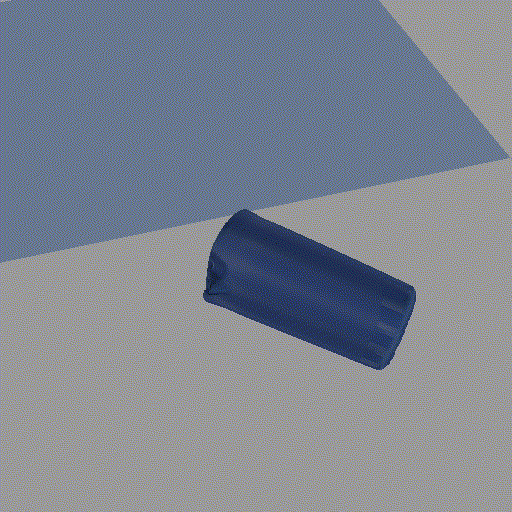
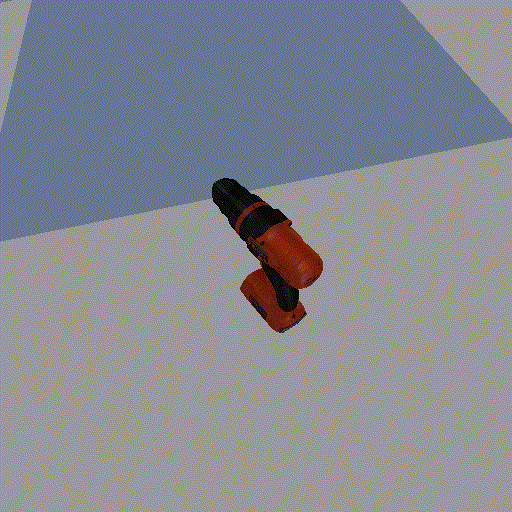
Successful refined grasping trajectories by residual RL, while replaying human demonstrations would fail due to tracking error and IK error.
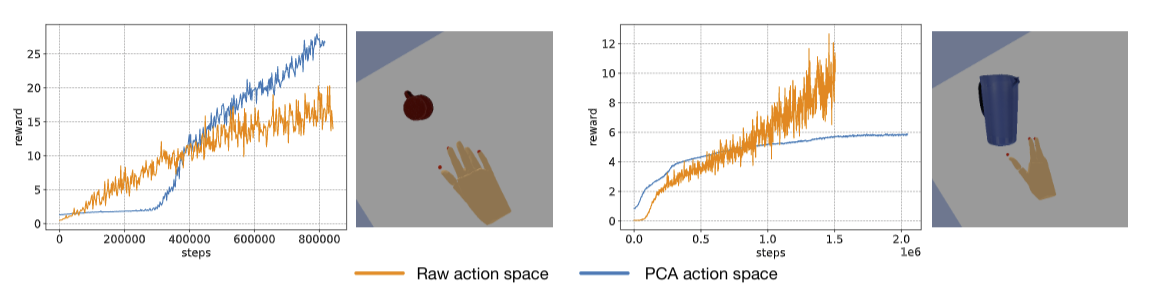
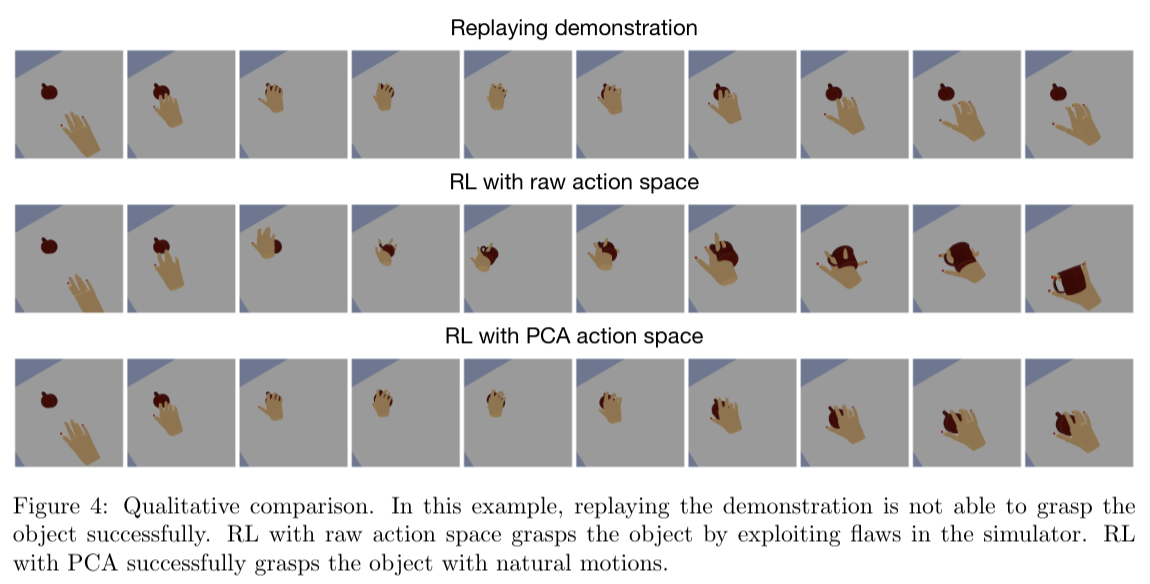